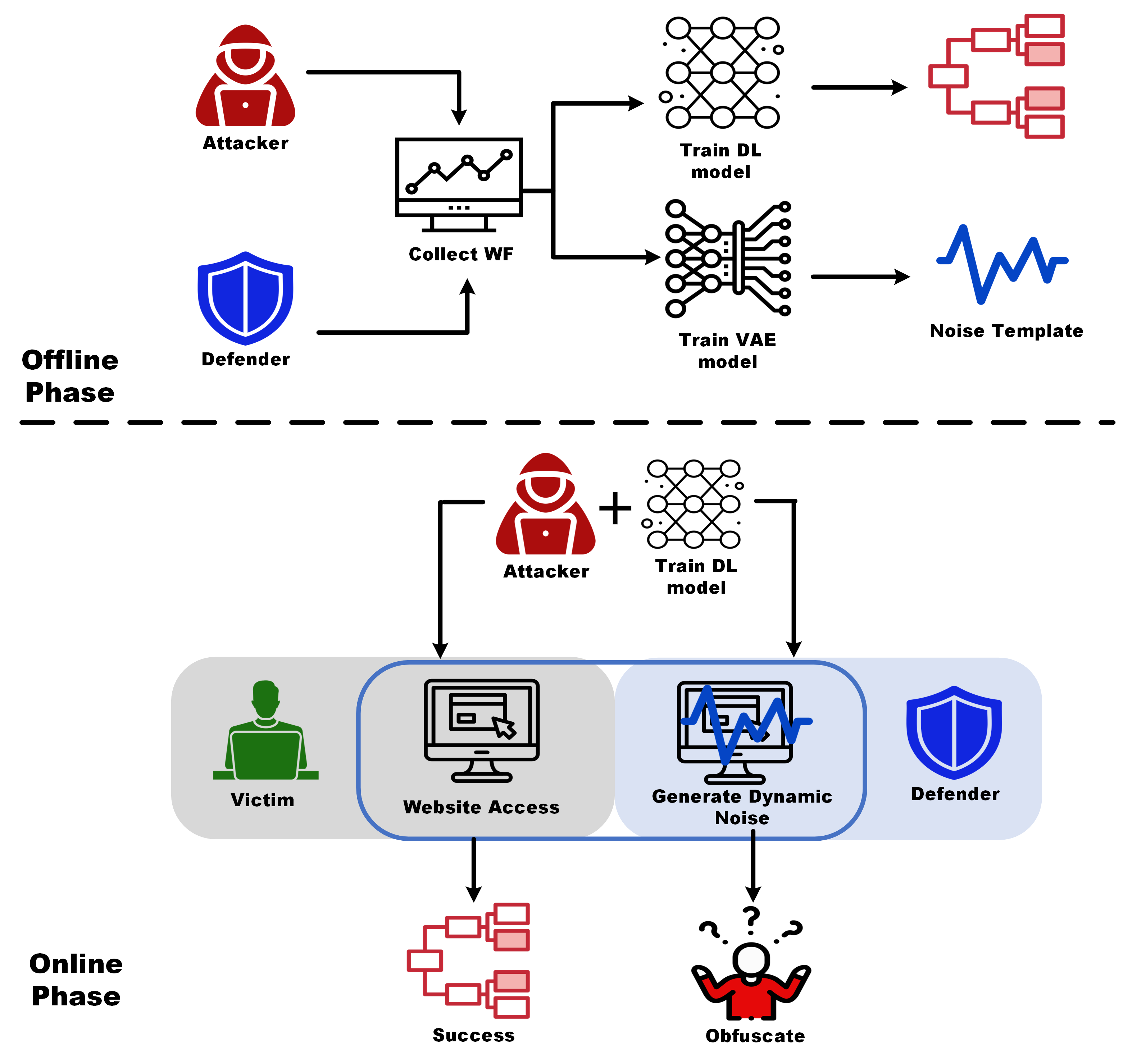
Abstract
Cache-based website fingerprinting (WF) attacks violate user privacy where the attacker leverages the shared last-level cache in CPUs and analyzes the fingerprints through machine learning and deep learning models. WF attacks are even applicable in Incognito and anonymized browser platforms, leading to a serious threat to the public. Several defense techniques inject random noise during website rendering to degrade the attack success rate, while these techniques either create large performance overhead or cannot obfuscate the WF dataset entirely when the attacker retrains a new learning model with noisy fingerprints.
In this work, we develop a dynamic generative learning-based defense technique, DefWeb, to protect user privacy against cache-based WF attacks by injecting precise noise into the WFs. For this purpose, (i) we train generative neural networks, Variational Autoencoder (VAE) to represent high-dimensional fingerprints in a low-dimension space while creating distinct clusters for each website. (ii) Minimal noise templates are extracted in the low-dimension space to obfuscate the fingerprints efficiently. (iii) We create practical noise templates that can be added to WFs during website rendering by leveraging Self-modifying Code (SMC). We implement DefWeb in simulation and real-world setups to degrade the attacker’s model accuracy. DefWeb can decrease the model accuracy to 1.1% and 28.8% in simulation and real-world setups, including Mozilla Firefox, Google Chrome, and Tor browsers. Finally, the performance overhead introduced by DefWeb is only 9.5%, considerably lower than previous defense techniques.
Installation
- Full version paper
- Download the DefWeb repo
- Read
README.md
file. - Demonstration version is available for testing